AI Image Recognition Guide for 2024
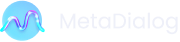
However, what is lost in such a simple operation is the freedom to create pictures. There are many such software available, and many people may be overwhelmed and not know how to choose a good and cheap or even free photo enhancer. Popular image recognition benchmark datasets include CIFAR, ImageNet, COCO, and Open Images. Though many of these datasets are used in academic research contexts, they aren’t always representative of images found in the wild. Researchers have developed a large-scale visual dictionary from a training set of neural network features to solve this challenging problem.

From physical imprints on paper to translucent text and symbols seen on digital photos today, they’ve evolved throughout history. Manually reviewing this volume of USG is unrealistic and would cause large bottlenecks of content queued for release. Google Photos already employs this functionality, helping users organize photos by places, objects within those photos, people, and more—all without requiring any manual tagging. Despite being 50 to 500X smaller than AlexNet (depending on the level of compression), SqueezeNet achieves similar levels of accuracy as AlexNet. This feat is possible thanks to a combination of residual-like layer blocks and careful attention to the size and shape of convolutions.
The tool performs image search recognition using the photo of a plant with image-matching software to query the results against an online database. When it comes to image recognition, Python is the programming language of choice for most data scientists and computer vision engineers. It supports a huge number of libraries specifically designed for AI workflows – including image detection and recognition. Image Detection is the task of taking an image as input and finding various objects within it. An example is face detection, where algorithms aim to find face patterns in images (see the example below). When we strictly deal with detection, we do not care whether the detected objects are significant in any way.
Often referred to as “image classification” or “image labeling”, this core task is a foundational component in solving many computer vision-based machine learning problems. The conventional computer vision approach to image recognition is a sequence (computer vision pipeline) of image filtering, image segmentation, feature extraction, and rule-based classification. Image Recognition AI is the task of identifying objects of interest within an image and recognizing which category the image belongs to.
Industry is facing increasing pressure to account for the content its products make. Experts are calling on the industry to prevent users from generating misleading and malicious material — and to offer ways of tracing its origin and distribution. Finding the right balance between imperceptibility and robustness to image ai that can identify images manipulations is difficult. Highly visible watermarks, often added as a layer with a name or logo across the top of an image, also present aesthetic challenges for creative or commercial purposes. Likewise, some previously developed imperceptible watermarks can be lost through simple editing techniques like resizing.
Despite the size, VGG architectures remain a popular choice for server-side computer vision models due to their usefulness in transfer learning. VGG architectures have also been found to learn hierarchical elements of images like texture and content, making them popular choices for training style transfer models. At viso.ai, we power Viso Suite, an image recognition machine learning software platform that helps industry leaders implement all their AI vision applications dramatically faster with no-code. We provide an enterprise-grade solution and software infrastructure used by industry leaders to deliver and maintain robust real-time image recognition systems.
Identifying AI-generated images with SynthID
SqueezeNet is a great choice for anyone training a model with limited compute resources or for deployment on embedded or edge devices. Visual recognition technology is widely used in the medical industry to make computers understand images that are routinely acquired throughout the course of treatment. Medical image analysis is becoming a highly profitable subset of artificial intelligence. In the future, they want to enhance the model so it can better capture fine details of the objects in an image, which would boost the accuracy of their approach.
Image recognition is an application of computer vision that often requires more than one computer vision task, such as object detection, image identification, and image classification. Since SynthID’s watermark is embedded in the pixels of an image, it’s compatible with other image identification approaches that are based on metadata, and remains detectable even when metadata is lost. The detection tool works well on DALL-E 3 images because OpenAI added “tamper-resistant” metadata to all of the content created by its latest AI image model. This metadata follows the “widely used standard for digital content certification” set by the Coalition for Content Provenance and Authenticity (C2PA). When its forthcoming video generator Sora is released the same metadata system, which has been likened to a food nutrition label, will be on every video. The first category is to use professional photo editing software like Adobe Photoshop or Luminar Neo.
There is no doubt that Photoshop is the most professional of all image edit software. You can foun additiona information about ai customer service and artificial intelligence and NLP. It has more features than any other photo editor, allowing you to edit your images with unlimited creativity. Photoshop can do almost everything from removing scratches, scuffs, and stains to improving the complexion, straightening hair, and whitening teeth. As powerful as it is, the use of the various buttons and the custom parameter settings is certainly a very complex and daunting task for someone who has not specifically learned how to use this software. Well, of course, as one of the most professional and widely used editing software, you can find many tutorials online, if you don’t mind such a huge learning curve and its expensive subscription fees.
- We provide an enterprise-grade solution and software infrastructure used by industry leaders to deliver and maintain robust real-time image recognition systems.
- As with many tasks that rely on human intuition and experimentation, however, someone eventually asked if a machine could do it better.
- On the other hand, image recognition is the task of identifying the objects of interest within an image and recognizing which category or class they belong to.
- The first method is for those who are highly specialized and good at using professional editing software, the second one is better for restoring photos that are not in good shape and need a lot of work.
- To ensure that the content being submitted from users across the country actually contains reviews of pizza, the One Bite team turned to on-device image recognition to help automate the content moderation process.
It seems that the C2PA standard, which was initially not made for AI images, may offer the best way of finding the provenance of images. The Leica M11-P became the first camera in the world to have the technology baked into the camera and other camera manufacturers are following suit. The image classifier will only be released to selected testers as they try and improve the algorithm before it is released to the wider public. The program generates binary true or false responses to whether an image has been AI-generated.
New type of watermark for AI images
In this way, some paths through the network are deep while others are not, making the training process much more stable over all. The most common variant of ResNet is ResNet50, containing 50 layers, but larger variants can have over 100 layers. The residual blocks have also made their way into many other architectures that don’t explicitly bear the ResNet name. The success of AlexNet and VGGNet opened the floodgates of deep learning research. As architectures got larger and networks got deeper, however, problems started to arise during training. When networks got too deep, training could become unstable and break down completely.
Before the researchers could develop an AI method to learn how to select similar materials, they had to overcome a few hurdles. First, no existing dataset contained materials that were labeled finely enough to train their machine-learning model. The researchers rendered their own synthetic dataset of indoor scenes, which included 50,000 images and more than 16,000 materials randomly applied to each object. Some tools, like Hive Moderation and Illuminarty, can identify the probable AI model used for image generation. No, while these tools are trained on large datasets and use advanced algorithms to analyze images, they’re not infallible. There may be cases where they produce inaccurate results or fail to detect certain AI-generated images.
breakdown of detected generators
As a reminder, image recognition is also commonly referred to as image classification or image labeling. To ensure that the content being submitted from users across the country actually contains reviews of pizza, the One Bite team turned to on-device image recognition to help automate the content moderation process. To submit a review, users must take and submit an accompanying photo of their pie. Any irregularities (or any images that don’t include a pizza) are then passed along for human review. Using a deep learning approach to image recognition allows retailers to more efficiently understand the content and context of these images, thus allowing for the return of highly-personalized and responsive lists of related results.
New OpenAI Tool Can Detect Dall-E 3 AI Images With 98% Accuracy – ExtremeTech
New OpenAI Tool Can Detect Dall-E 3 AI Images With 98% Accuracy.
Posted: Wed, 08 May 2024 11:00:00 GMT [source]
For more inspiration, check out our tutorial for recreating Dominos “Points for Pies” image recognition app on iOS. And if you need help implementing image recognition on-device, reach out and we’ll help you get started. The benefits of using image recognition aren’t limited to applications that run on servers or in the cloud. Many of the most dynamic social media and content sharing communities exist because of reliable and authentic streams of user-generated content (USG). But when a high volume of USG is a necessary component of a given platform or community, a particular challenge presents itself—verifying and moderating that content to ensure it adheres to platform/community standards. With modern smartphone camera technology, it’s become incredibly easy and fast to snap countless photos and capture high-quality videos.
This occurs when a model is trained on synthetic data, but it fails when tested on real-world data that can be very different from the training set. The approach can also be used for videos; once the user identifies a pixel in the first frame, the model can identify objects made from the same material throughout the rest of the video. As experts warn that images, audio and video generated by artificial intelligence could influence the fall elections, OpenAI is releasing a tool designed to detect content created by its own popular image generator, DALL-E. Start-up acknowledges that this tool is only a small part of what will be needed to fight so-called deepfakes in the months and years to come. AI-generated images have become increasingly sophisticated, making it harder than ever to distinguish between real and artificial content. AI image detection tools have emerged as valuable assets in this landscape, helping users distinguish between human-made and AI-generated images.
Multiclass models typically output a confidence score for each possible class, describing the probability that the image belongs to that class. Image-based plant identification has seen rapid development and is already used in research and nature management use cases. A recent research paper analyzed the identification accuracy of image identification to determine plant family, growth forms, lifeforms, and regional frequency.
In the case of single-class image recognition, we get a single prediction by choosing the label with the highest confidence score. In the case of multi-class recognition, final labels are assigned only if the confidence score for each label is over a particular threshold. Currently, convolutional neural networks (CNNs) such as ResNet and VGG are state-of-the-art neural networks for image recognition. In current computer vision research, Vision Transformers (ViT) have recently been used for Image Recognition tasks and have shown promising results. Other face recognition-related tasks involve face image identification, face recognition, and face verification, which involves vision processing methods to find and match a detected face with images of faces in a database.

A custom model for image recognition is an ML model that has been specifically designed for a specific image recognition task. This can involve using custom algorithms or modifications to existing algorithms to improve their performance on images (e.g., model retraining). However, engineering such pipelines requires deep expertise in image processing and computer vision, a lot of development time and testing, with manual parameter tweaking.
The Inception architecture solves this problem by introducing a block of layers that approximates these dense connections with more sparse, computationally-efficient calculations. Inception networks were able to achieve comparable accuracy to VGG using only one tenth the number of parameters. There are a few steps that are at the backbone of how image recognition systems work. Although two objects may look similar, they can have different material properties. OpenAI previously added content credentials to image metadata from the Coalition of Content Provenance and Authority (C2PA).
For example, you may have a dataset of images that is very different from the standard datasets that current image recognition models are trained on. In this case, a custom model can be used to better learn the features of your data and improve performance. Alternatively, you may be working on a new application where current image recognition models do not achieve the required accuracy or performance. AlexNet, named after its creator, was a deep neural network that won the ImageNet classification challenge in 2012 by a huge margin. The network, however, is relatively large, with over 60 million parameters and many internal connections, thanks to dense layers that make the network quite slow to run in practice. In general, deep learning architectures suitable for image recognition are based on variations of convolutional neural networks (CNNs).
As with many tasks that rely on human intuition and experimentation, however, someone eventually asked if a machine could do it better. Neural architecture search (NAS) uses optimization techniques to automate the process of neural network design. Given a goal (e.g model accuracy) and constraints (network size or runtime), these methods rearrange composible blocks of layers to form new architectures never before tested. Though NAS has found new architectures that beat out their human-designed peers, the process is incredibly computationally expensive, as each new variant needs to be trained.
SynthID isn’t foolproof against extreme image manipulations, but it does provide a promising technical approach for empowering people and organisations to work with AI-generated content responsibly. This tool could also evolve alongside other AI models and modalities beyond imagery such as audio, video, and text. We’re committed to connecting people with high-quality information, and upholding trust between creators and users across society. Part of this responsibility is giving users more advanced tools for identifying AI-generated images so their images — and even some edited versions — can be identified at a later date. Both the image classifier and the audio watermarking signal are still being refined.
The introduction of deep learning, in combination with powerful AI hardware and GPUs, enabled great breakthroughs in the field of image recognition. With deep learning, image classification and deep neural network face recognition algorithms achieve above-human-level performance and real-time object detection. AI Image recognition is a computer vision task that works to identify and categorize various elements of images and/or videos. Image recognition models are trained to take an image as input and output one or more labels describing the image. Along with a predicted class, image recognition models may also output a confidence score related to how certain the model is that an image belongs to a class.
Recognition of the images with artificial intelligence includes train and tests based on Python. We hope the above overview was helpful in understanding the basics of image recognition and how it can be used in the real world. Results indicate high AI recognition accuracy, where 79.6% of the 542 species in about 1500 photos were correctly identified, while the plant family was correctly identified for 95% of the species. “The user just clicks one pixel and then the model will automatically select all regions that have the same material,” he says. Images for download on the MIT News office website are made available to non-commercial entities, press and the general public under a
Creative Commons Attribution Non-Commercial No Derivatives license. A credit line must be used when reproducing images; if one is not provided
below, credit the images to “MIT.”
In image recognition, the use of Convolutional Neural Networks (CNN) is also called Deep Image Recognition. However, deep learning requires manual labeling of data to annotate good and bad samples, a process called image annotation. The process of learning from data that is labeled by humans is called supervised learning. The process of creating such labeled data to train AI models requires time-consuming human work, for example, to label images and annotate standard traffic situations for autonomous vehicles. The terms image recognition and computer vision are often used interchangeably but are different.
These approaches need to be robust and adaptable as generative models advance and expand to other mediums. This tool provides three confidence levels for interpreting the results of watermark identification. If a digital watermark is detected, part of the image is likely generated by Imagen.
During experiments, the researchers found that their model could predict regions of an image that contained the same material more accurately than other methods. When they measured how well the prediction compared to ground truth, meaning the actual areas of the image that are comprised of the same material, their model matched up with about 92 percent accuracy. Since the model is outputting a similarity score for each pixel, the user can fine-tune the results by setting a threshold, such as 90 percent similarity, and receive a map of the image with those regions highlighted.
OpenAI’s new tool can detect its own DALL-E 3 AI images, but there’s a catch – ZDNet
OpenAI’s new tool can detect its own DALL-E 3 AI images, but there’s a catch.
Posted: Tue, 07 May 2024 14:12:00 GMT [source]
One of the most popular and open-source software libraries to build AI face recognition applications is named DeepFace, which is able to analyze images and videos. To learn more about facial analysis with AI and video recognition, I recommend checking out our article about Deep Face Recognition. Existing methods for material selection struggle to accurately identify all pixels representing the same material. For instance, some methods focus on entire objects, but one object can be composed of multiple materials, like a chair with wooden arms and a leather seat. Other methods may utilize a predetermined set of materials, but these often have broad labels like “wood,” despite the fact that there are thousands of varieties of wood. The method is accurate even when objects have varying shapes and sizes, and the machine-learning model they developed isn’t tricked by shadows or lighting conditions that can make the same material appear different.
In general, traditional computer vision and pixel-based image recognition systems are very limited when it comes to scalability or the ability to re-use them in varying scenarios/locations. SynthID contributes to the broad suite of approaches for identifying digital content. One of the most widely used methods of identifying content is through metadata, which provides information such as who created it and when.

While generative AI can unlock huge creative potential, it also presents new risks, like enabling creators to spread false information — both intentionally or unintentionally. Being able to identify AI-generated content is critical to empowering people with knowledge of when they’re interacting with generated media, and for helping prevent the spread of misinformation. AI detection will always be free, but we offer additional features as a monthly subscription to sustain the service. We provide a separate service for communities and enterprises, please contact us if you would like an arrangement. Now that we know a bit about what image recognition is, the distinctions between different types of image recognition, and what it can be used for, let’s explore in more depth how it actually works. Of course, this isn’t an exhaustive list, but it includes some of the primary ways in which image recognition is shaping our future.
Encoders are made up of blocks of layers that learn statistical patterns in the pixels of images that correspond to the labels they’re attempting to predict. High performing encoder designs featuring many narrowing blocks stacked on top of each other provide the “deep” in “deep neural networks”. The specific arrangement of these blocks and different layer types they’re constructed from will be covered in later sections.
Likewise, Luminar Neo is more versatile and flexible in terms of freedom, but it’s not for beginners either. For example, there are multiple works regarding the identification of melanoma, a deadly skin cancer. Deep learning image recognition software allows tumor Chat PG monitoring across time, for example, to detect abnormalities in breast cancer scans. To overcome those limits of pure-cloud solutions, recent image recognition trends focus on extending the cloud by leveraging Edge Computing with on-device machine learning.
The goal of image detection is only to distinguish one object from another to determine how many distinct entities are present within the picture. “In machine learning, when you are using a neural network, usually it is learning the representation and the process of solving the task together. The pretrained model gives us the representation, then our neural network just focuses on solving the task,” he says.
SynthID allows Vertex AI customers to create AI-generated images responsibly and to identify them with confidence. While this technology isn’t perfect, our internal testing shows that it’s accurate against many common image manipulations. From brand loyalty, to user engagement and retention, and beyond, implementing image recognition on-device has https://chat.openai.com/ the potential to delight users in new and lasting ways, all while reducing cloud costs and keeping user data private. And because there’s a need for real-time processing and usability in areas without reliable internet connections, these apps (and others like it) rely on on-device image recognition to create authentically accessible experiences.
Today, in partnership with Google Cloud, we’re launching a beta version of SynthID, a tool for watermarking and identifying AI-generated images. This technology embeds a digital watermark directly into the pixels of an image, making it imperceptible to the human eye, but detectable for identification. Similarly, apps like Aipoly and Seeing AI employ AI-powered image recognition tools that help users find common objects, translate text into speech, describe scenes, and more. Two years after AlexNet, researchers from the Visual Geometry Group (VGG) at Oxford University developed a new neural network architecture dubbed VGGNet. VGGNet has more convolution blocks than AlexNet, making it “deeper”, and it comes in 16 and 19 layer varieties, referred to as VGG16 and VGG19, respectively.
Some photo recognition tools for social media even aim to quantify levels of perceived attractiveness with a score. In all industries, AI image recognition technology is becoming increasingly imperative. Its applications provide economic value in industries such as healthcare, retail, security, agriculture, and many more. To see an extensive list of computer vision and image recognition applications, I recommend exploring our list of the Most Popular Computer Vision Applications today.
AI Image recognition is a computer vision technique that allows machines to interpret and categorize what they “see” in images or videos. Action localization identifies and localizes human actions within video sequences, making them searchable, analyzable, and more meaningful. For more details on platform-specific implementations, several well-written articles on the internet take you step-by-step through the process of setting up an environment for AI on your machine or on your Colab that you can use. A lightweight, edge-optimized variant of YOLO called Tiny YOLO can process a video at up to 244 fps or 1 image at 4 ms.
Google Cloud is the first cloud provider to offer a tool for creating AI-generated images responsibly and identifying them with confidence. This technology is grounded in our approach to developing and deploying responsible AI, and was developed by Google DeepMind and refined in partnership with Google Research. AVC.AI is an advanced online tool that uses artificial intelligence to improve the quality of digital photos. It is able to automatically detect and correct various common photo problems, such as poor lighting, low contrast, and blurry images. The results are often dramatic, and can greatly improve the overall look of a photo, and the results can be previewed in real-time, so you can see exactly how the AI is improving your photo. This final section will provide a series of organized resources to help you take the next step in learning all there is to know about image recognition.
RCNNs draw bounding boxes around a proposed set of points on the image, some of which may be overlapping. Single Shot Detectors (SSD) discretize this concept by dividing the image up into default bounding boxes in the form of a grid over different aspect ratios. Viso Suite is the all-in-one solution for teams to build, deliver, scale computer vision applications. “We wanted a dataset where each individual type of material is marked independently,” Sharma says.
Agricultural machine learning image recognition systems use novel techniques that have been trained to detect the type of animal and its actions. AI image recognition software is used for animal monitoring in farming, where livestock can be monitored remotely for disease detection, anomaly detection, compliance with animal welfare guidelines, industrial automation, and more. If you don’t want to start from scratch and use pre-configured infrastructure, you might want to check out our computer vision platform Viso Suite. The enterprise suite provides the popular open-source image recognition software out of the box, with over 60 of the best pre-trained models.
- Photoshop can do almost everything from removing scratches, scuffs, and stains to improving the complexion, straightening hair, and whitening teeth.
- Images for download on the MIT News office website are made available to non-commercial entities, press and the general public under a
Creative Commons Attribution Non-Commercial No Derivatives license.
- But the company said the tool was not designed to detect images produced by other popular generators like Midjourney and Stability.
In a year stacked with major elections around the world, calls for ways to monitor the lineage of A.I. In recent months, audio and imagery have already affected political campaigning and voting in places including Slovakia, Taiwan and India. Because this kind of deepfake detector is driven by probabilities, it can never be perfect. So, like many other companies, nonprofits and academic labs, OpenAI is working to fight the problem in other ways. Start-up is also joining an industrywide effort to spot content made with artificial intelligence. Generative AI technologies are rapidly evolving, and computer generated imagery, also known as ‘synthetic imagery’, is becoming harder to distinguish from those that have not been created by an AI system.
It also provides data collection, image labeling, and deployment to edge devices – everything out-of-the-box and with no-code capabilities. Creating a custom model based on a specific dataset can be a complex task, and requires high-quality data collection and image annotation. Explore our article about how to assess the performance of machine learning models.
While early methods required enormous amounts of training data, newer deep learning methods only needed tens of learning samples. SynthID uses two deep learning models — for watermarking and identifying — that have been trained together on a diverse set of images. The combined model is optimised on a range of objectives, including correctly identifying watermarked content and improving imperceptibility by visually aligning the watermark to the original content. Deep learning image recognition of different types of food is applied for computer-aided dietary assessment. Therefore, image recognition software applications have been developed to improve the accuracy of current measurements of dietary intake by analyzing the food images captured by mobile devices and shared on social media. Hence, an image recognizer app is used to perform online pattern recognition in images uploaded by students.
Later in this article, we will cover the best-performing deep learning algorithms and AI models for image recognition. While computer vision APIs can be used to process individual images, Edge AI systems are used to perform video recognition tasks in real-time, by moving machine learning in close proximity to the data source (Edge Intelligence). This allows real-time AI image processing as visual data is processed without data-offloading (uploading data to the cloud), allowing higher inference performance and robustness required for production-grade systems. An Image Recognition API such as TensorFlow’s Object Detection API is a powerful tool for developers to quickly build and deploy image recognition software if the use case allows data offloading (sending visuals to a cloud server). The use of an API for image recognition is used to retrieve information about the image itself (image classification or image identification) or contained objects (object detection). While pre-trained models provide robust algorithms trained on millions of datapoints, there are many reasons why you might want to create a custom model for image recognition.